The Importance of Data Analytics in SaaS Product Development
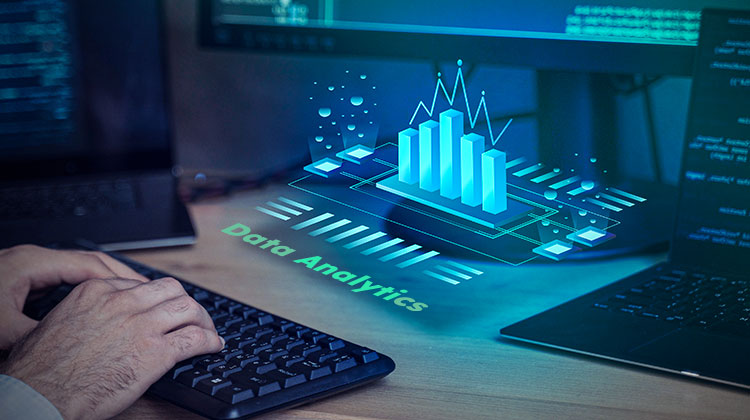
Software as a Service (SaaS) providers strive to develop products that meet current market demands while simultaneously trying to anticipate future needs.
This persistent struggle demands a strategic use of SaaS data analytics.
A data-driven approach to SaaS development allows providers to make more informed decisions about every aspect of their product.
Data analysis helps businesses decide which new features to include, what changes the user interface needs, and how to improve customer support services and the overall user experience.
It ensures that decision-making is grounded in concrete, actionable data, not based on intuition alone.
In short, strategic data analysis leads to more successful products and a stronger competitive edge in the market.
Role of Data Analytics in Product Development
Data analytics provides a quantitative foundation upon which companies can make logical decisions about which features to introduce, enhance, or retire.
By analyzing user engagement data, companies can identify which features are most popular and which are underperforming.
Regarding product design, data analytics can reveal patterns in how users interact with a SaaS application. Heat maps, for instance, can show which areas of an application receive the most attention, while clickstream analysis can reveal the journey users typically take through an app.
When it comes to user experience (UX) and user interface (UI) improvements, analytics is a gold mine. It helps in understanding user behavior, preferences, and pain points. For instance, if analytics reveal that users frequently abandon a particular process, it may indicate a design flaw or a need for more intuitive navigation.
By addressing these issues, companies can enhance user satisfaction, leading to increased engagement and retention.
Enhancing Customer Insights Through Data
Data-driven understanding is essential for creating a customer-centric product strategy.
Customer Insights
The process begins with data collection from various touchpoints, such as user activity logs, customer support interactions, feedback forms, and social media engagements.
Segmentation is a key tactic here, where customers are divided into groups based on common characteristics or behaviors, allowing for more targeted analysis.
Data analytics enables companies to move beyond basic demographic information to psychographic and behavioral insights. This deeper insight can reveal why customers behave in certain ways, what motivates them, their level of product engagement, and even their likelihood to churn.
Patterns and Behavior
Identifying patterns within the data can uncover trends that take time to become visible. For instance, usage patterns can indicate the most popular times of day for product use, which features are most and least engaged with, and the typical user journey within the application.
Behavior analysis can predict future actions of customers, like upgrades or cancellations, based on their product usage history.
Personalized User Experiences
When SaaS providers understand their users’ behavior and preferences, they can tailor the user experience to meet individual needs.
Personalization drives up the product’s perceived value, as users are likelier to feel that the software is “made for them.” This can be personalized dashboards, recommended actions, or alerts specific to each user’s use patterns.
This improves retention rates and can turn users into advocates for the product, as they have experiences worth sharing.
Data-Driven Decision Making
Companies reduce guesswork and biases that often cloud judgment by relying on data.
Feature Prioritization
A SaaS company might use a SaaS analytics platform to track feature usage across its user base.
Suppose the data reveals that a particular feature is used by a high percentage of the most profitable customers. In that case, the company may prioritize enhancements to that feature over others.
Customer Churn Reduction
By analyzing churn rates and correlating them with specific features or user behaviors, a company can identify at-risk customers.
Interventions can then be developed to retain those customers, such as targeted outreach or offering incentives to continue using the service.
Market Expansion
Data analytics can reveal geographic or industry-specific trends in product usage.
For instance, if users from a particular region or industry are showing higher engagement rates, a SaaS company might decide to focus its marketing efforts or even customize the product for that segment.
Pricing Strategy
SaaS businesses often use data analytics to optimize their pricing models. The company can adjust its strategy to maximize revenue without losing users by analyzing how different customer segments respond to pricing changes.
Customer Support Improvements
Analytics can help identify common issues users face, allowing for proactive improvements in customer support resources.
For example, if data shows that many support tickets are related to a specific problem, the company might create a tutorial or a feature update to address this issue.
Product Roadmap Adjustments
Data from user feedback and product performance can influence the long-term strategy of a product’s development.
If analytics show a shift in market needs or user preferences, the company might re-evaluate its product roadmap to stay relevant and competitive.
Operational Efficiency and Performance Monitoring
Data analytics can bring about efficiencies by optimizing processes, reducing waste, and enhancing productivity.
Process Optimization
Data analytics can reveal inefficiencies in a SaaS company’s internal processes. Companies can identify bottlenecks and areas where processes can be automated or improved by analyzing workflows.
For example, data might show that the manual onboarding process for new customers is time-consuming and prone to errors. By automating this process, a company can reduce the time to onboard new users and free up staff to focus on more critical tasks.
Resource Allocation
Analytics can guide decision-makers on where to allocate resources most effectively. Data on user engagement can inform which product areas need more attention and development.
If data shows that a particular feature drives a lot of support tickets, resources can be allocated to improve that feature or to provide better training for the support team.
Predictive Maintenance
In SaaS, predictive maintenance can refer to anticipating and addressing service issues before they impact customers.
Data analytics can predict system outages or performance degradation, allowing for preventive maintenance and thus reducing downtime.
Performance Tracking
KPIs are the lifeblood of performance monitoring, providing measurable values demonstrating how effectively a company achieves key business objectives.
Data analytics enables tracking these indicators, offering insights into the health of various operations.
Common KPIs in SaaS include monthly recurring revenue, churn rate, customer acquisition cost, and customer lifetime value.
Real-Time Monitoring
Real-time data monitoring is critical in a SaaS environment due to the fast-paced nature of the industry.
It allows companies to react immediately to any changes in user behavior or system performance.
For instance, a sudden drop in user activity might indicate a problem with the product that needs immediate attention.
Alerting and Notifications
Automated alerting systems can notify relevant stakeholders when certain KPIs fall below or exceed predetermined thresholds. This can help in averting crises or capitalizing on opportunities as they arise.
For example, when a business runs a promotional campaign, it might deliver an unexpected spike in new user sign-ups. An automated alert to the customer service team helps them prepare for the increased volume of inquiries and onboarding.
Common Challenges in Integrating Data Analytics
Data Quality and Integrity
One of the primary challenges is ensuring the quality and accuracy of data. Poor data quality can lead to misleading analytics results. SaaS providers must establish robust data collection and processing methodologies to maintain the integrity of their data.
Data Volume and Management
The sheer volume of data that needs to be processed can be overwhelming. Efficient data management strategies are essential to handle, store, and process large datasets without compromising performance.
Skill Set and Resource Allocation
Data analytics requires a specific skill set, including data science, machine learning, and statistical analysis. SaaS companies often face the challenge of acquiring the right talent or training their existing workforce to meet these needs.
Integration with Existing Systems
Integrating analytics capabilities into existing SaaS products can be technically challenging. It requires a seamless blend of the analytics engine with the core functionalities of the product, often necessitating significant architectural changes.
Real-Time Analysis
Providing real-time or near-real-time data analytics can be technically complex and resource-intensive, yet it’s increasingly becoming a user expectation.
Data Privacy
With the increasing emphasis on user privacy, SaaS providers must navigate complex data privacy laws such as GDPR and CCPA. They need to ensure that user data is collected, processed, and stored in compliance with these regulations, which often require explicit user consent and clear data usage policies.
Security Concerns
Ensuring the security of data is paramount. Data breaches can lead to significant financial and reputational damage. SaaS companies must invest in robust security measures, including encryption, access controls, and regular security audits, to protect user data.
Ethical Use of Data
The ethical implications of data analytics cannot be overlooked. This includes concerns around user consent, transparency in data usage, and avoiding biases in data analysis and AI models. SaaS companies must establish ethical guidelines for data usage and ensure that their analytics tools do not inadvertently lead to unfair or discriminatory outcomes.
User Trust and Transparency
Maintaining user trust is critical. This involves being transparent about data collection and use practices. Users should be informed about what data is being collected, how it is being used, and what benefits they receive in return.
Compliance and Regulatory Challenges
Navigating the evolving landscape of data protection laws and regulations is an ongoing challenge. Compliance must be continually assessed and updated as laws change, and new regulations are introduced.
Data Analytics: The Key to SaaS Development
The continuous influx of data and the advancements in analytics tools provide an unparalleled opportunity for SaaS products to become more responsive, efficient, and user-centric.
SaaS developers and companies are at a pivotal moment where leveraging data analytics user-centric not just beneficial but essential for success. It is an invitation to embrace the power of data to transform their products and services.
By integrating data analytics into their development processes, SaaS companies can achieve new levels of innovation, customer satisfaction, and operational efficiency.